Enterprises Must Now Rework Their Knowledge into AI-Ready Forms: Vector Databases and LLMs
April 10, 2024 Leave a comment
With the recent arrival of potent new AI-based models for representing knowledge, the methods enterprises use to manage data today is now faced with yet another major new transformation. I remember a few decades back when the arrival of SQL databases were a major innovation. At the time, they were both quite costly and took great skill to use well. Despite this, enterprises readily understood they were the best new game in town in which their most important data had to live, and so move they did.
Now vector databases and especially foundation/large language models (LLMs) have shifted the focus — in just a couple of short years — on the way organizations must now store and retrieve their own data . And we are also right back at the beginning of the maturity curve that most of us left behind a couple of decades ago.
While not everyone realizes this yet, the writing is now on the wall: Much of our business data now has to migrate again and be recontextualized into these new models. Because the organizations that don’t will likely be at a significant disadvantage, given what AI models of our data can deliver in terms of value.
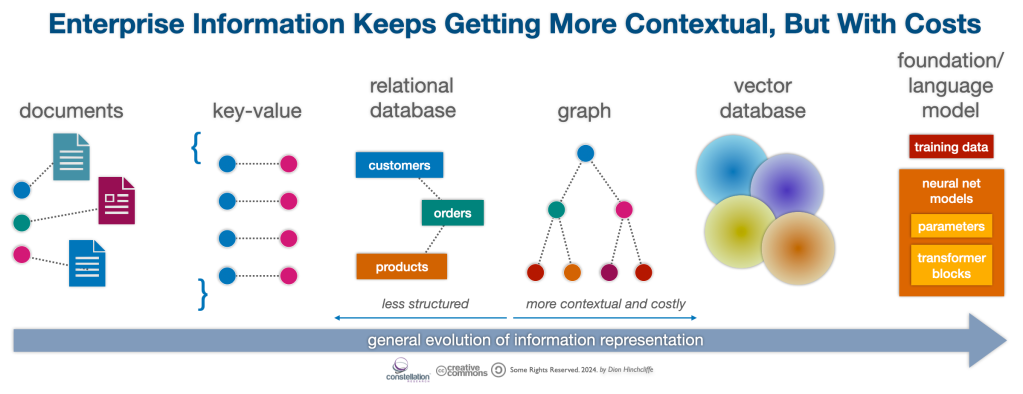
Our Marathon with Organizational Data Will Continue With AI
The result, like a lot of technology disruption, will be an journey through a series of key stages of maturation. Each one will progressively enrich the way our organizations store, understand, and leverage our vast reservoirs of information using AI. This process will naturally be somewhat painful, and not all data will need to migrate. And certainly, our older database models aren’t going anywhere either. But at the core of this shift will be the creation of our own private AI models of organizational knowledge. These models must be carefully developed, nurtured, and protected, while also made highly accessible, with appropriate security models.
We’ve moved on from the early days of digital documents, capturing loosely structured data in primitive forms, to the highly structured revolutions introduced by relational and graph databases. Both phases marked a significant movement forward in how data is conceptualized and utilized within the enterprise. The subsequent emergence of JSON as a lightweight, text-based lingua franca further bridged the gap between these two worlds and the burgeoning Web, offering a structured yet flexible way to represent data that catered to the needs of modern Internet applications/services and also helped give rise to NoSQL, a mini-boom of a new database model that ultimately found a home in many Internet-based systems, but largely didn’t disrupt our businesses like AI will.
AI-Models Are A Distinct Conceptual Shift in Working with Data
However, the latest advancements in knowledge representation really do usher in a steep increase in technical sophistication and complexity. Vector databases and foundation models, including large language models (LLMs), represent a genuine quantum leap in how enterprises can manage their data, introducing unprecedented levels of semantic insight, contextual understanding, and universal access to knowledge. Such AI models are able to find and understand the hidden patterns that tie diverse datasets together. This ability can’t be understated and is a key attribute that emerges from a successful model training process. As such, it is one of the signature breakthroughs of generative AI.
Let’s go back to the unknown issues with AI in the enterprise. This uncertainty ranges from what the more effective technical and operational approaches are to picking the best tools/platforms and supporting vendors. This new vector- and model-based era is characterized by an exponential increase in not just the sophistication with which data is stored and interpreted, but in the very way it is vectorized, tokenized, embedded, trained on, represented and transformed. Each of these requires a separate set of skills and understanding, and very considerable compute resources. While this can be outsourced to some degree, this has many risks of its own, not least is that such outsourcers may not deeply understanding the domain of the business and how best to translate it into an AI model.

AI-Based Technologies for Enterprise Data
Vector databases, leveraging the power of machine learning to deeply understand and query enterprise data in ways that mimic human cognitive processes, offered us the first new glimpse into a new future. Going forward, the contextual understanding of our data will largely be based on these radical new forms that bear little resemblance to what came before. Similarly, foundation models like LLMs have revolutionized information management by providing tools that can seemingly comprehend, generate, synthesize, and interact with human language in a manner using neural nets, vast pre-trained parameter sets, and complex transformer blocks that each have a high learning curve to set up and create (using them, however, is very easy.) These technologies provide us with a new dawn of possibilities, from enhanced decision-making processes with unparalleled insights using all our available knowledge, to automating complex tasks with a nuanced understanding of language and context. But all these new AI technologies are generally not familiar to IT departments, which now have to make strategic sense of them for the organization.
Thus, this remarkable progress brings with it a large number of concerns and hurdles to make reality. First, the creation, deployment, and utilization of these sophisticated data models — at least with current technologies — entrails significantly higher costs compared to previous approaches to representing data, according to HBR. A real-world cost example: Google has a useful AI pricing page for benchmarking fundamental costs, which breaks down the various cloud-based AI rates, with grounding requests costing $35 per 1K requests,. Grounding — the process of ensuring that the output of an AI is factually correct — is probably necessary for many types of business scenarios using AI, and is thus a significant extra cost not required in other types of data management systems.
Furthermore, the computational resources, available time, and time required to develop and maintain such systems are also quite substantial. Moreover, the transition to these advanced data management solutions involves navigating a complex landscape of technical, organizational, and ethical considerations.
Related: How to Embark on the Transformation of Work with Artificial Intelligence
As enterprises stand on the cusp of this major new migration to AI, the journey ahead promises real rewards. It also demands careful strategizing, intelligent adoption, and I would argue at this early date, a lot of experimentation, prototyping, and validation. The phases of integrating vector databases and foundation models into the fabric of enterprise knowledge management will require a nuanced approach backed by rigorous testing, balancing the potential for transformative improvements against the practicalities of implementation costs and the readiness of organizational infrastructures to support such advancements.
That this is already happening, there is little doubt, based on my conversations with IT leaders around the world. We are witnessing the beginning of a significant shift in how enterprise knowledge is stored, accessed, and utilized. This transition, while demanding serious talent development and capability acquisition, offers an opportunity to redefine the very boundaries of what is possible in data management and utilization. The key to navigating this evolution lies in a strategic, informed approach to adopting these powerful new models, ensuring that an enterprise can harness its full potential while mitigating the risks and costs associated with such groundbreaking technological advancements.
Early Approaches For Private AI Models of Enterprise Data
Right now, the question I’m most often asked about enterprise AI is how best to create private AI models. Given the extensive concerns that organizations currently have about losing intellectual property, protecting customer/employee/partner privacy, complying with regulations, and giving up control over the irreplaceable asset of enterprise data to cloud vendors, there is a lot of searching around for workable approaches that produce cost-effective private AI models that produce results while minimizing the potential downsides and risks of AI.
As part of my current research agenda on generative AI strategy for the CIO, I’ve identified a number of initial services and solutions from the market to help with creating, operating, and managing private AI models. Each has their own pros and cons.
Services to Create Private AI Models of Enterprise Data
PrivateLLM.AI – This service will train an AI model on your enterprise data and host it privately for exclusive use. They specialize in a number of vertical and functional domains including legal, healthcare, financial services, government, marketing, and advertising.
Turing’s LLM Training Service – Trains large language models (LLMs) for enterprises. Turing uses a variety of techniques to improve the LLMs they create, including data analysis, coding, and multimodal reasoning. They also offer speciality AI services like supervised fine-tuning, reinforcement learning from human feedback (RLHF), and direct preference optimization (DPO), which helps optimizing language models to adhere to human preferences.
LlamaIndex – A popular way to connect LLMs to enterprise data. Has hundreds of connectors to common applications and impressive community metrics (700 contributors with 5K+ apps created.) Enables use of many commercial LLMs, so must be carefully evaluated for control and privacy issues. Make it very easy to use Retrieval-Augmented Generation (RAG), a way to combine a vector database of enterprise information with pass-through to a LLM for targeted but highly enriched results, and even has a dedicated RAG offering to make it easy.
Gradient AI Development Lab – This is an end-to-end service for creating private LLMs. They offer LLM strategy, model selection, training and fine-tuning services to create custom AIs. They specialize in high security AI models and offer SOC2, GDPR, and HIPAA certifications and guarantees enterprise data “never leaves their hands.”
Datasaur.AI – They offer an LLM creation service that provides customized models for LLM development including using vector stores to provide enterprise-grade domain-specific context. They offer a wide choice of existing commercial LLMs to build on as well, so care must be taken to create a private LLM instance. They are more platform-based than some of the others, which makes it easier to get started, but may limit customization downstream.
Signity – Has a private LLM development service that is optimized more to specific data science applications.However, they can handle the whole LLM development process, from designing the model architecture, developing the model and then tuning it. They can create custom models using PyTorch, TensorFlow and many other popular frameworks.
TrainMy.AI – A service that enables enterprises to run an LLM on a private server using retrieval augmented generation (RAG) for enterprise content. While it is more aimed at chatbot and customer service scenarios, it’s very easy to use and allows organizations to bring in vectorized enterprise data for RAG enhancement into a conversational AI service that is entirely controlled privately.
NVIDIA NeMo – For creating serious enterprise-grade custom models, NVIDIA, the GPU industry leader and leading provider of AI chips, offers an end-to-end “compete” solution to creating enterprise LLMs. From model evaluation to AI guardrails, the platform is very rich and is ready to use, if you can come up with the requisite GPUs.
Clarifai – Offers a service that enterprise can quickly use for AI model training. It’s somewhat self-service and allows organization to set up models quickly and continually learn from production data. Has pay-as-you-go pricing and can train pre-built, pre-optimized models of their own already pre-trained with millions of expertly labeled inputs, or you can build your own model.
Hyperscaler LLM Offerings – If you trust your enterprise data to commercial clouds and want to run your own private models in them, that is possible too and all the major cloud vendors offer such capabilities including AWS’s SageMaker JumpStart, Azure Machine Learning, and Google Cloud offers private model training on Vertex AI. These are more for IT departments wanting to roll their own AI models and don’t produce business-ready results without technical experience, unlike many of the services listed above.
Cerebras AI Model Services – The maker of the world’s largest AI chip also offers large-scale private LLM training. They take a more rigorous approach with a team of PhD researchers and ML engineers that they report will meticulously prepare experiments and quality assurance checks to ensure a predictable AI model creation journey to achieve desired outcomes.
Note: If you want to appear on this list, please send me a short description to dion@constrellationr.com.
Build or Customize an LLM: The Major Fork in the Road
Many organizations, especially those unable to maintain sufficient internal AI resources, will have to decide whether to build their own AI model of enterprise data or carefully use a third party service. The choice will be tricky. For example, OpenAI now offers a fine-tuning service, for example, that allows enterprise data to augment how GPT-3.5 or 4 produces domain specific data. This is a slippery slope, as there are many advantages to building on a high capability model, but many risks, including losing control over valuable IP.
Currently, I believe that the cost of training private LLMs will continue to fall steadily, and that service bureaus will increasingly make it turn-key for anyone to create capable AI models while preserving control and privacy. The reality is, that most enterprises will have a growing percentage of their knowledge stored and accessed in AI-ready formats, and the ones that move their most strategic and high value data early are likely to be the most competitive in the long run. Will vector databases and LLMs become the dominant model for enterprise knowledge? The jury is still out, but I believe they will almost certainly become about as important as SQL databases are today. But the main point is clear: It is high time for most organizations to proactively cultivate their AI data-readiness.
My Related Research
AI is Changing Cloud Workloads, Here’s How CIOs Can Prepare
A Roadmap to Generative AI at Work
Spatial Computing and AI: Competing Inflection Points
Salesforce AI Features: Implications for IT and AI Adopters
Video: I explore Enterprise AI and Model Governance
Analysis: Microsoft’s AI and Copilot Announcements for the Digital Workplace
How Generative AI Has Supercharged the Future of Work
How Chatbots and Artificial Intelligence Are Evolving the Digital/Social Experience
The Rise of the 4th Platform: Pervasive Community, Data, Devices, and AI