How to Embark on the Transformation of Work with Artificial Intelligence
August 4, 2023 Leave a comment
As we stand at the threshold of our AI-powered future, it’s now clear that artificial intelligence is no longer merely a tantalizing possibility, it’s a practical necessity in the workplace already. Leaders in all sectors are recognizing that AI has the power to transform not only their business but their industry. Yet, as with any technological innovation, deploying AI to the workforce isn’t as simple as flipping a switch. AI in particular will be a nuanced, multifaceted endeavor that requires real understanding of the issues and sustained, adequate preparation.
One of the most important starting points in this journey is understanding the journey itself. To do this, I’ve begun assembling an early map of the AI transformation of work, which I lay out below. It’s a work in progress but substantially what most organizations will have to go through over the next few years. Most organizations won’t realize all of these moving parts as a single effort, but instead as a series of efforts over the years that will increasingly connect and integrate.
Understanding the Path Towards Responsible Use of AI for Work
Today, creating a capable foundation for AI for the workforce is no longer a luxury; it’s an essential step towards proper integration of the technology to our work. Now, we must craft thoughtful and robust policies and strategies that align AI with our broader organizational goals. This includes setting data and tech standards that ensure quality, consistency, and ethical use of AI. Without these foundational elements, our over-hasty attempts to harness the power of AI can lead to significant challenges, inefficiency, and undesired consequences. Therefore, taking a more methodical and strategic approach is not just wise, it’s indispensable.
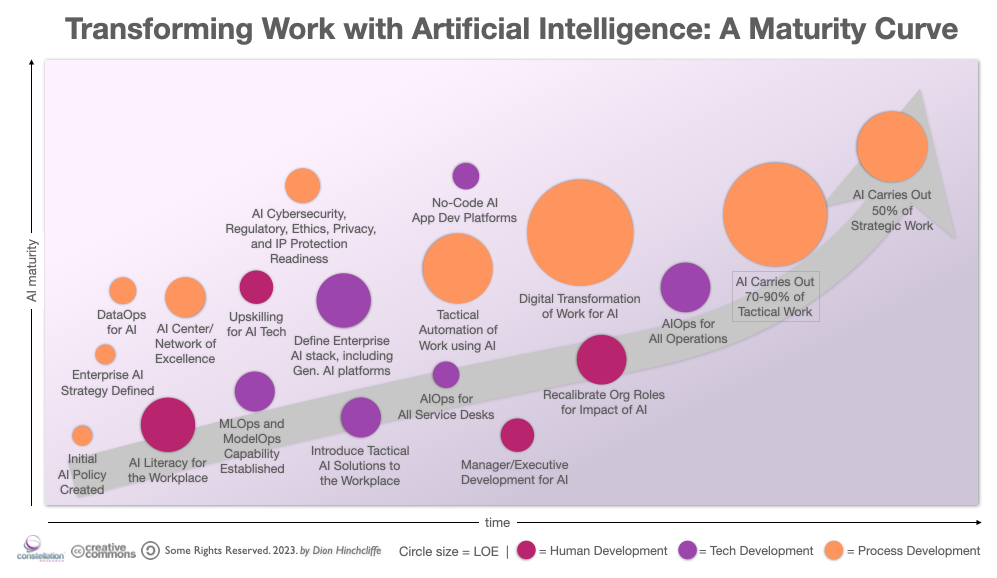
One of the questions I get asked most often these days is what transforming work with AI looks like. In the figure above, I depict the key elements for an enterprise-wide effort to do this. This is an early take and as I mentioned, bound to change, but it’s about as accurate as we can determine right now, in terms of the moving parts across people, technology, and process.
This maturity curve for AI work transformation will be turned into a living artifact that I’ll be developing into a more in-depth research effort soon. However, I break down the key components here so that we can have an basic industry vocabulary and understanding of what must be done to realize AI properly in the workplace over the next 3-5 years, which this maturity curve roughly covers.
The Key Activities for Transforming Work with AI
Here are what I currently believe should be the top activities — from the maturity curve show above — and what each activity represents:
Initial AI Policy. An enterprise-class AI policy is far more than a mere set of guidelines. It’s a vital blueprint that governs how artificial intelligence will function, integrate, and evolve within our organizations. A well-crafted AI policy will include, at its core, a clear articulation of the ethical principles guiding the use of AI, ensuring alignment with both societal norms and legal requirements. It will define roles and responsibilities for AI governance, creating accountability and oversight. To facilitate compliance and quality control, standardized procedures and methodologies (like ModelOps, discussed below) will be laid out. This includes comprehensive data management practices, addressing everything from security to privacy to the integrity and bias of data. The policy must also encompass risk management strategies to identify and mitigate potential pitfalls and unwanted outcomes. Perhaps most crucially, an enterprise-class AI policy will embed mechanisms for continuous learning and adaptation by the workforce. AI is a very rapidly changing field. Thus, the policy must be agile enough to evolve, ensuring that it remains relevant and effective. Such a comprehensive policy isn’t merely a bureaucratic necessity; it’s a strategic imperative and must be regularly updated. By clearly defining the rules of the game, it creates a safe and fertile ground for innovation, collaboration, and growth, positioning the enterprise to fully leverage the transformative power of AI.
AI Strategy for the Enterprise. An AI strategy stands as a compass, helping navigate the complex and often turbulent waters of innovation using cognitive technologies. A robust business and tech-focused AI strategy must work in harmony, resonating with the broader business goals while leveraging the cutting-edge capabilities of AI tech. At its core, the strategy will align AI initiatives with business objectives, ensuring that each investment and every project, is targeted towards tangible outcomes and measurable ROI. It will sketch a clear roadmap, outlining the technology infrastructure, skills development, partnerships, and investments needed to bring AI to life within the organization. But more than a roadmap, it’s also a adaptable framework, that links technological advancements to needed market shifts. It must delineate ethical guidelines, ensuring that AI is developed and deployed responsibly. It must also embrace innovation, fostering a culture that encourages experimentation and learning. Perhaps most essentially, it should be interwoven with a clear understanding of customer/stakeholder needs, how AI will meet those needs, and add value in meaningful new ways. This isn’t merely a plan, it’s a promise, a commitment to integrating AI into the fabric of the organization, leveraging it as a tool, a partner, and a catalyst for growth and transformation. Every worker should be familiar with the strategy at a high-level at least.
DataOps for AI. This capability represents a critical operational shift in how we approach data management and integration within the internal AI landscape of a business. It’s not only a methodology but a cultural transformation that brings agility, quality, and collaboration together as a key practice. By creating a seamless conduit between data scientists, engineers, and business stakeholders, DataOps streamlines the flow of data, ensuring that it’s readily accessible, trustworthy, and aligned with business goals. It’s the oil in the AI machine, reducing friction and accelerating innovation. The result is enhanced AI capabilities, reduced time-to-value, and a more responsive, adaptable, and higher quality approach to leveraging AI.
AI Literacy Program for the Workforce. AI literacy for the workforce is no longer confined to the realm of technologists. It’s a universal workforce imperative. Several facets are key: AI literacy that weaves together technological comprehension with ethical responsibility. It’s not just understanding algorithms; it’s about grasping the ‘why’ and ‘how’ behind AI decisions, known as explainability. Workers must have the tools to interrogate AI, to ask not only what it’s doing but why it’s doing it, ensuring alignment with human values and corporate policies. Ethical responsibilities loom large, requiring a proper appreciation of the potential biases that can inadvertently be built into AI systems. The ability to identify, challenge, and remove these biases is a key skill, reflecting a commitment to fairness and equity. Understanding and following corporate AI policy becomes not merely a rule but a cultural norm, integrating the safe and responsible use of AI into the fabric of organizational life. But AI literacy is more than a set of skills. It’s a mindset, a recognition that every worker, regardless of role or rank, must be empowered to engage with AI, to understand it, question it, and harness it. This is not just about staying ahead of the curve. It’s about defining the curve, transforming AI from just a tool into a business partner, and leveraging it to create a more intelligent, ethical, and innovative future. Such literacy programs will be delivered using today’s advanced learning technologies.

Establish an AI Center/Network of Excellence. The establishment of an AI Center of Excellence (CoE) is an essential milestone in the journey of AI adoption, embodying a central hub of knowledge, innovation, and excellence. The AI CoE acts as the organizational nerve center, driving AI strategy, governance, best practices, resource kits, project advisory, and collaboration. It houses experts and practitioners who not only understand the intricacies of AI but also have the vision to align it with the overarching business goals. The CoE ensures that AI is not a disparate set of projects but an integrated, strategic initiative that permeates every aspect of the organization. However, the pervasive nature of AI, its broad impact across all functions and levels, demands a more nuanced approach. A Network of Excellence, a more decentralized version of the CoE that I’ve developed and proven out over the years, may be more apt. It recognizes that AI excellence doesn’t reside in a single point but is a collective endeavor, a shared responsibility. By fostering collaboration and knowledge-sharing across various units and teams, a Network of Excellence democratizes AI, making it accessible, relevant, and responsive to the unique needs and opportunities of different parts of the organization. This isn’t just a structural adjustment; it’s a philosophical shift, a recognition that the power of AI lies in its integration into the fabric of the organization, facilitated by a network that is as dynamic, diverse, and decentralized as the technology itself.
Realizing MLOps and ModelOps for AI. The burgeoning disciplines of MLOps and ModelOps stand as pivotal frameworks for AI. They essentially sculpt the chaos of AI being used ad hoc in every corner of the org into a more coherent effort. MLOps, or Machine Learning Operations, integrates the world of machine learning with DevOps principles, orchestrating a seamless flow from development to deployment to monitoring. It’s about creating a collaborative environment where data scientists, engineers, and operations professionals converge, ensuring that machine learning models are not just created but effectively implemented and continuously optimized. ModelOps takes this a step further, focusing on the lifecycle management of AI and machine learning models, ensuring that they remain relevant, accurate, and aligned with business objectives, including vitally, cost efficiency. Together, MLOps and ModelOps embody a systematic, enterprise-wide approach, turning the art of AI into a precise science. In an age where AI is not a mere accessory but a core business driver, the disciplined, structured approach of MLOps and ModelOps is not really an option; it’s a necessity and now best practice. It’s the operational architecture that allows AI to live, to adapt, and to thrive, transforming it from a tool into a strategic partner.
Video: How ModelOps is Essential to Enterprise-Wide AI Governance
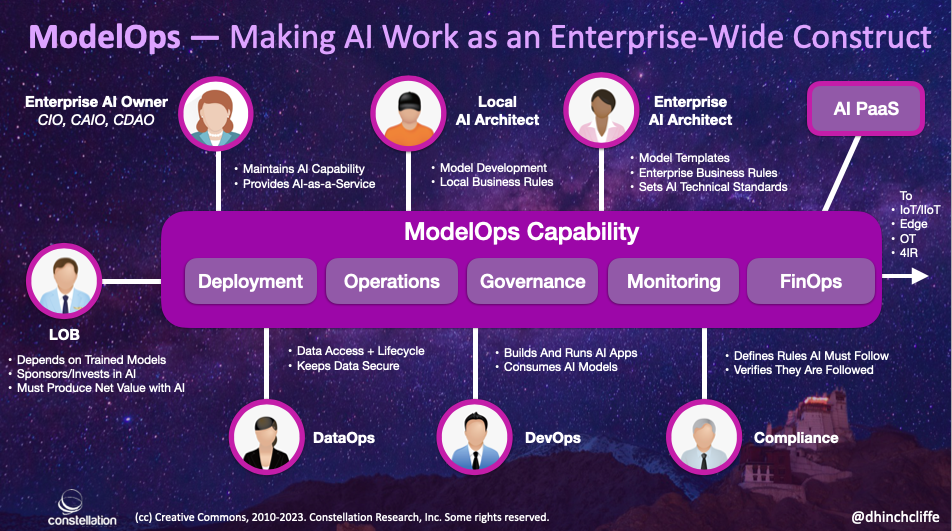
Upskilling Technical Workers on AI. In the competitive and turbulent waters of AI talent acquisition, organizations are facing a stark reality: the pursuit of external AI expertise is a race that is both exhausting and expensive. That’s why upskilling existing technical workers on AI tech emerges not merely as an alternative but often as a superior strategy. It leverages an existing understanding of the organization’s culture, goals, and systems, embedding AI expertise within a context of institutional knowledge. Upskilling fosters a sense of growth and loyalty, turning current employees into AI champions who are invested in the organization’s success. It’s a recognition that the seeds of innovation often reside within, waiting for the nourishment of knowledge and opportunity. In an era where AI talent is a precious commodity, upskilling is not just a path to empowerment. It can be a strategic imperative, transforming existing resources into a source of innovation and growth.
Cybersecurity, Regulatory Compliance, Ethics, Privacy, and IP Protection for AI Readiness. Embracing AI is not just a technological endeavor. It’s a complex journey that binds together the strands of cybersecurity, regulatory compliance, ethics, privacy, and intellectual property protection. As a cross check of policy and governance — especially if it is being operationalized properly — organizations must craft a more holistic AI readiness strategy, one that recognizes AI’s transformative power while also respecting its intricate risks and responsibilities. This involves creating a robust framework that aligns AI with legal obligations, ethical principles, and societal expectations. It means forging a culture where security is not an afterthought but an integral part of AI design and deployment. It demands transparency, accountability, and a relentless commitment to protecting both individual privacy and intellectual property. This isn’t merely about compliance; it’s about trust. Building readiness for these crucial aspects is not just a safeguard; it’s a covenant with customers, employees, and stakeholders, a promise that the organization’s journey with AI will be responsible, respectful, and reliable.
Establish an AI technology Stack, Including Generative AI. In the diverse and dynamic landscape of AI, consistency and robustness are not just incidental features. An organization’s enterprise AI stack must be a carefully constructed edifice, where each layer and every component is aligned and harmonized. This includes the vital inclusion of generative AI platforms, which open the door to creativity, innovation, and customization at an unprecedented scale. A consistent and robust AI stack ensures that the various elements of AI, from data processing to modeling to deployment, work in concert, not conflict. It creates a shared language and a unified framework, removing silos and fostering collaboration across teams and functions. Generative AI platforms add a layer of adaptability, enabling the organization to create new foundation models, solutions, and experiences, tailored to specific needs and opportunities. This isn’t merely about efficiency, it’s about agility and relevance. In an environment where change is constant and disruption is the norm, a well-defined, consistent, and robust enterprise AI stack reduces unnecessary duplication, inconsistency, and time-to-value. It’s the strong and scalable backbone that supports the organization’s AI ambitions, ensuring that they are not just experiments but efforts that are sustainable, scalable, and impactful initiatives from the outset.
Introduce Tactical AI Solutions to the Workplace. Rolling out generative AI to the workforce, especially when leveraging 3rd party applications, is a combined effort of innovation and control, creativity and compliance. It begins with alignment with internal corporate information, creating bridges between 3rd party tools and proprietary data, ensuring that they speak the same language but also adhere to the same rules, especially security and customer privacy. Protecting Intellectual Property (IP) in these systems is a key goal, and thus, robust security protocols and clear usage guidelines must be woven into the fabric of the integration. The effort continues with a thoughtful measurement of Return on Investment (ROI), crafting KPIs that capture not only the tangible efficiencies and savings but also the sometime hard to measure qualities of agility, innovation, and user satisfaction. Providing workers with clear, actionable guidance is essential. They must not only understand how to use the tools but also how to leverage them to save time, innovate, and add value. Attribution to AI, recognizing and celebrating where and how it contributes, fosters a culture of transparency and trust. This isn’t only a technical rollout. It’s a cultural transformation, a strategic alignment of technology, people, and purpose. It’s about turning generative AI into a useful partner, empowering the workforce to explore, create, and excel, all within a framework that safeguards the organization’s values, assets, and integrity.
Related Research: How Generative AI Work Apps are Supercharging the Future of Work
AIOps for All Service Desk Functions. The transformative wave of AIOps (Artificial Intelligence for Operations) is not merely a technological shift; it’s a harbinger of a new era of efficiency and responsiveness. Typically one of the first systematic operational transformations that leverage AI, AI is revolutionizing service desk activities across domains like IT and HR. By infusing intelligence into operations, it’s turning mundane tasks into automated workflows and complex problems into AI-powered service solutions. It’s providing real-time insights, predictive analytics, and intelligent automation, making service desks not just reactive but proactive. It’s a reimagining of what service desks can be, transforming them from cost centers into value generators, from problem solvers into drivers of innovation, as the AI service desk can steadily improve itself over time.
Tactical Automation of Work. Once enough of a foundational has been built to move more quickly and comprehensively with AI automation, businesses will embarking on the systematic automation of work using AI. It will be a multifaceted endeavor, filled with promise and complexity in equal measure. For an enterprise, this journey begins with the rigorous mapping of workflows using tools like process mining, identifying areas ripe for automation, where AI can add value without diluting quality or humanity. It’s about striking a balance, harnessing the power of AI to enhance efficiency and accuracy without losing the essence of creativity and empathy. Integrating AI into existing systems requires seamless orchestration, ensuring that automated workflows align with human processes, complementing rather than conflicting. Building robust, transparent AI models that adhere to ethical guidelines and legal compliance is paramount, weaving trust into the very fabric of automation. Monitoring, evaluating, and continuously refining AI-driven automation becomes an standard process and a commitment to excellence and adaptability. Systematic automation with AI is not a singular act. It’s done in concert with, a confluence of technology, strategy, ethics, culture, and vision. It’s a journey that requires planning, agility, and an ongoing commitment to transforming work, not just doing work. The goal is not merely to do more with less but to do better with more, leveraging AI to unlock potential, creativity, and growth.
No-Code AI App Development Platforms. The brand-new industry of AI-led application development tools is upending the traditional expectations of software engineering, turning app creation from an advanced human craft into a collaboration with an intelligent agent that can code. Initially, AI algorithms will help analyze requirements, user needs, and business goals to develop apps that are not just functional but intuitive. But the real revolution begins post-deployment. Conversational app development takes the reins, enabling to user to engage in continuous evolution, refinement, and enhancement of the app without the need for traditional coding skills. Users can interact with the AI app dev agent, ask for new features, report issues, and suggest capabilities through natural language. The app listens, learns, and adapts, adding features, refining capabilities, and fixing bugs. It’s an organic, dynamic process where the app grows not just with the users but because of the users. This unleashes a new wave of AI automation within organizations, democratizing app development, and turning it from a technical specialty into a shared endeavor. It fosters innovation, agility, and inclusiveness, allowing apps to be living entities that evolve, adapt, and innovate. AI-led application development is not merely a new technology; it’s a new philosophy, a recognition that the best apps are not built but grown, nurtured by the collective intelligence of AI and humanity. I’ll be releasing a new AI App Dev ShortList later this year to describe the companies I’m now seeing emerging to realize this vision.
Executives and Managers Adapt to AI. As work becomes steadily more automated and AI-enabled, managers and executives face a profound transformation themselves. The traditional skills of oversight, coordination, and control, though still relevant, must be infused with new capabilities and a new mindset that recognizes automation as a partner. Managers must evolve from being supervisors to being orchestrators, understanding how to leverage automation to enhance human capabilities, not replace them. It’s about recognizing the nuanced interplay between machine efficiency and human creativity, knowing when to automate and when to humanize. Executives must become visionaries, seeing beyond the immediate gains of cost and time savings, recognizing the broader strategic potential of automation. It’s about fostering a culture of innovation and agility, where automation is a catalyst for growth, a tool for exploration, a means to unleash potential. Adaptation will also involve understanding the ethics and responsibilities of automation, ensuring that decisions are not just driven by algorithms but guided by values and policy. Transparency, fairness, empathy – these must be woven into the fabric of the automated workplace. Learning to communicate with, interpret, and even question AI-driven insights will become a vital skill, turning data into wisdom, information into strategy. And perhaps most importantly, managers and executives must learn to lead in an environment where leadership is shared, where machines are not just tools but collaborators, where the wisdom of the crowd includes both human and artificial voices. In the automated workplace, being a manager or executive is a linchpin role. It’s not about wielding authority but inspiring collaboration between human and machine to explore possibilities. It’s a journey that requires courage, curiosity, and a willingness to redefine not just how we work but why we work.
Digital Transformation of Work. Digitally transforming work with AI will then go well beyond tactical automation; it’s an orchestration of technology, strategy, and vision, aimed at reinventing how work is done. For instance, in customer service, AI can create personalized, 24/7 support experiences, not just answering queries but predicting and pre-empting issues. In supply chain management, AI can transcend basic tracking and inventory control, employing predictive analytics to optimize logistics, reduce waste, and enhance sustainability. In human resources, AI’s role is not confined to sorting resumes but extends to identifying skill gaps, personalizing training, and fostering a culture of continuous learning and growth. It’s a journey from the mundane to the meaningful, from the mechanical to the magical, turning work from a task into a purpose, an opportunity not just to do more but to be more.
Recalibrate Org Roles for the Impact of AI. After the initial stages of an AI transformation of work within an organization, there will emerge a pivotal moment — and probably several such stages — where roles and responsibilities must be restructured and recalibrated. AI doesn’t merely change how tasks are performed; it alters the very nature of roles, turning them from fixed functions into fluid collaborations. Responsibilities shift from executing tasks to managing, interpreting, and innovating with AI. The hierarchy flattens, silos break down, and the organization evolves into a network of interconnected, AI-enhanced roles. It’s a reimagining of the workplace where titles give way to talents, where the emphasis is not on what you do but how you contribute. This restructuring is not a disruption. It’s an evolution, a necessary step in the journey from a traditional organization to a transformative, agile, AI-enabled enterprise.
AIOps for All Operations. AI operations are destined to expand beyond the boundaries of IT and HR, permeating all operational functions and processes within an organization. This is a recognition that AI’s potential is universal, its applicability almost certainly boundless. From marketing, where AI can personalize campaigns and predict trends, to manufacturing, where it can optimize production and enhance quality control, to finance, where it can manage risk and automate compliance, AI is quickly becoming the common thread that weaves through every function. It’s turning organizations into seamless, interconnected, intelligent entities, where data flows and insights are put to use automatically, where decisions are informed and agile, where innovation is not confined to departments but is a shared endeavor. Yet it is all still guided by humans. AI operations are not an add-on; they’re an ethos, a strategic alignment of technology and purpose that redefines what an organization can be and what it can achieve.
The Ongoing Journey of AI Transformation of Work
At some point, most tactical work and about half of strategic work will be completely automated after be re-imagined for an intelligent world. This is for now, as far as we can reliably see into the AI future. I’ll be working on fleshing this journey out in more detail, as our digital workplaces and employee experiences evolve and become much more AI-centric.
Please provide feedback in comments below or drop me an e-mail at dion@constellationr.com.
My Additional Related Research
The Future of Work in 2024: Navigating Through Uncertainties
An Analysis of Microsoft’s AI and Copilot Capabilities for the Digital Workplace
Every Worker is a Digital Artisan of Their Career Now
How to Think About and Prepare for Hybrid Work
Why Community Belongs at the Center of Today’s Remote Work Strategies
Reimagining the Post-Pandemic Employee Experience
It’s Time to Think About the Post-2020 Employee Experience
Research Report: Building a Next-Generation Employee Experience: 2021 and Beyond
The Crisis-Accelerated Digital Revolution of Work
Revisiting How to Cultivate Connected Organizations in an Age of Coronavirus
How Work Will Evolve in a Digital Post-Pandemic Society
A Checklist for a Modern Core Digital Workplace and/or Intranet
Creating the Modern Digital Workplace and Employee Experience
The Challenging State of Employee Experience and Digital Workplace Today
The Most Vital Hybrid Work Management Skill: Network Leadership